The period right after the Dartmouth Conference from 1956 until about 1974 was defined as the first summer of Artificial Intelligence. Thanks to the progress achieved, researchers were very optimistic about the future of AI and computers performed an increasing amount of operations, from English-language dialogue to solving algebraic equations.
Despite funding for AI research, at that time computers were not yet able to process and store enough data and information. The AI had greater difficulties in the very areas that seemed simpler, such as machine translation or reproduction of natural language. For example, an English language analysis program could handle a vocabulary of only 20 words.
The financiers realized that the search yielded insufficient results and withdrew their support: thus, began the first winter of the AI that would last until the early 1980s.
Expert Systems
The first AI programs were written in such a way as to arrive at the solution of a problem by “reasoning” through a series of logical propositions. It was in the early 1980s that a different approach emerged: knowledge-based systems.
Also called expert systems, these artificially reproduce the performance of an experienced person in a given field of knowledge or subject. An expert system is therefore a computer program that, after having been properly instructed by a professional, is able to deduce information from a set of data and starting information.
There are different types according to the type of problem addressed (interpretation, recognition, diagnosis) and its characteristics.
Following an analysis of a number of facts or circumstances and by inductive or deductive processes, an expert system is able to reach a conclusion and to solve particularly complex problems even without the intervention of a second expert who has specific skills on the subject.
Expert systems are therefore modeled on human knowledge, and behave like consultants: an example may be that of an expert system that suggests and gives advice to a general practitioner about diagnosis and therapy to be adopted with a patient.
DENDRAL, first Expert System
The first example of an expert system was DENDRAL (acronym for the term “DENDRitic ALgorithm”), developed in 1965 by Edward Feigenbaum, also referred to as the “father of expert systems”, and by Joshua Lederberg at Stanford University in California.
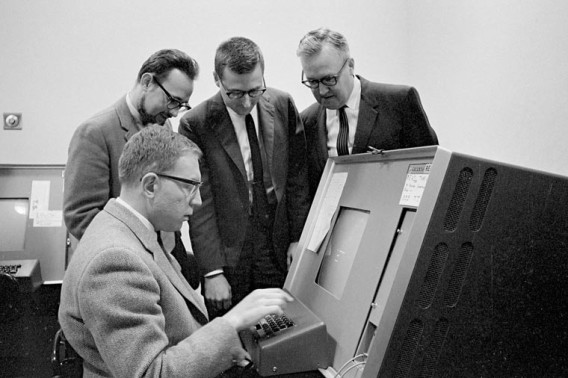
The task of this program was to map the structure of molecules, to help chemists identify unknown organic molecules. By analyzing the spectral analysis of the molecule on a rule-based basis, DENDRAL defined a set of possible structures; it then compared these to the data to determine which one was correct. DENDRAL program is considered the first expert system because it has allowed to automate decision-making and problem-solving behavior of organic chemists.
MYCIN, another expert system derived from DENDRAL, was instead thought of as a tool to help doctors in the diagnosis of infectious blood diseases. It focused on identifying the bacteria that caused infections and recommending antibiotics.
These early expert systems did not solve generic problems, rather they exploited boolean logic (in which variables can only take true or false values) and logical reasoning according to a deterministic model of cause-effect. Despite being machines that seemed to analyze and “think”, the human expert was still far superior to the artificial expert system.
The 1980s and second generation Expert-Systems
In the 1980s interest grew around the application of expert systems, projects and experiments multiplied. In this way, expert systems of “second generation” were born: the probabilistic model that reasoned on the causes and the possible effects was introduced.
This intense period of development was also favored by the application that expert systems had in the industrial and commercial field. The first implementation that had significant economic success was R1 (or Xcon, eXpert CONfigurer). Developed at Carnegie-Mellon University by John Mc Dermott in 1978, it was introduced in 1982 by the Digital Equipment Corporation to configure computer orders and improve their accuracy: based on customer orders, R1 was able to ensure that the order was complete but also to determine the spatial relations between the components (the system had more than 100 components with various possibilities of interaction). Four years later, the company was able to save $40 million a year.
Thanks to this renewed success, new systems based on knowledge and knowledge engineering were born. Japan was the first nation to invest heavily in computers designed for AI, so America, United Kingdom, and the rest of Europe followed suit.
However, even second-generation expert systems had problems. Above all, the difficulty in writing the rules that reflected the knowledge of the experts, and the management and maintenance of the same. In addition, the clamor around expert systems was growing much faster than the technological maturity of the time.
The enthusiasm then led to disappointment. Apple and IBM introduced more powerful general-purpose computers than those designed for AI, destroying the Artificial Intelligence industry. In addition, in 1987 DARPA, the government agency of the United States Department of Defense and one of the major funders of research on Artificial Intelligence (in 1985 alone had spent $ 100 million for research in the field) decided to stop investing by choosing to focus on technologies with better prospects in the short term.
Once again, investment, trust and the study of Artificial Intelligence suffered another setback, a situation that will last until the mid-1990s. Another AI winter was coming and enthusiasm for expert systems was fading.
The return of Neural Networks
Since their inception in the 1940s, neural networks have fascinated the scientific community. Artificial neural networks are mathematical models composed of artificial neurons inspired by biological neural networks (human or animal) and are used to solve engineering problems related to different technological fields such as computer science, electronics, simulation or other disciplines.
Opinions about neural networks have not always been positive. In 1969 Marvin Minsky and Seymour Papert, in their article “Perceptrons“, defined neural networks as inadequate for solving real problems and for any practical application.
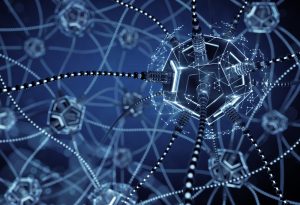
In the mid-1980s, however, there was a rediscovery of neural networks and the algorithm of “back-propagation” was reinvented, initially conceived in 1969 by Bryson and Ho, related to learning (inherent in both computer science and psychology) for neural networks.
This algorithm made it possible to create an alternative to symbolic models (used by McCarthy, Newell and Simon and many others) thanks to connectionist models, which aim to explain the functioning of the mind by using artificial neural networks. These models, just like the previous ones, were not able to create a real scientific progress.
As a result, models based on a more than alternative connessionist approach were seen as complementary to those using a symbolic approach.
Find more articles on the fascinating history of Artificial Intelligence under the category AIhistory or at this link.